Unlock the Value of Data Science: Make or Break?
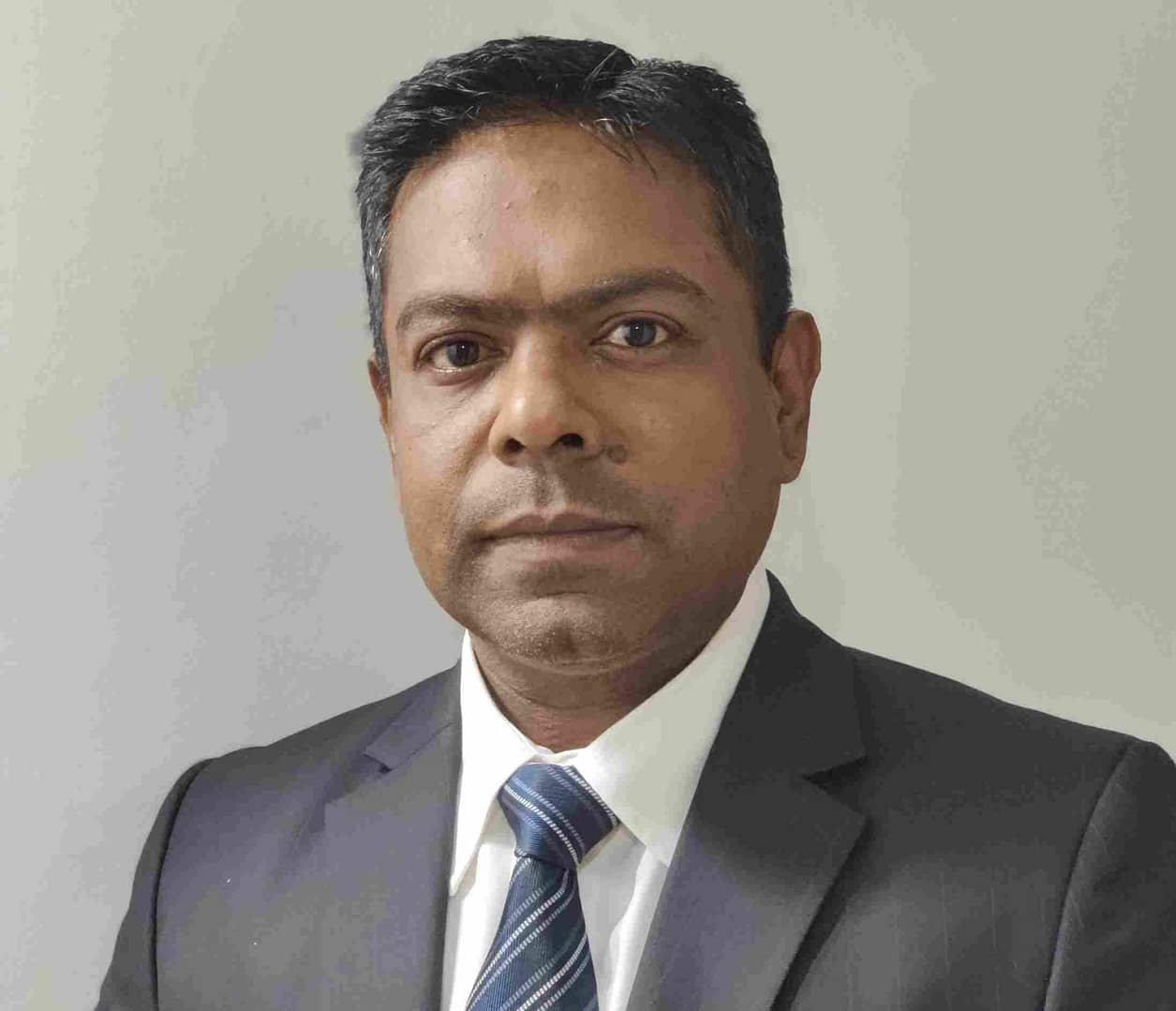
AUTHOR: TUSHAR BELAPURKAR
SENIOR ASSOCIATE CONSULTANT
Tushar is a Senior Operational Excellence professional with 20+ years of global experience. He specialises in Business & Digital Transformation and Continuous Improvement, with particular expertise in Data Science, Process Automation, Business & Function Process Architecture Design & Deploy, Shared-Service Set-Up and Migrations.
2022: A Make or Break Year?
Gartner has sounded the alarm, declaring 2022 a make-or-break year for CFOs in Data Science for Finance. The time for action is now.
2022 will be a make-or-break year for CFOs when it comes to unlocking the value of AI, hyper-automation, digital skills, continuous processes and data management. CFOs who continue to drive toward the future of autonomous Finance will unlock immense value for their organisation in the years to come.
Garter for Finance: Top Priorities for Finance Leaders in 2022
©2022 Gartner
So, it is probably time to start if you aren’t already planning to develop and digitise your organisation’s processes, data, tools, and skills.
And, at Loughridge Transformations, we would have to agree. We certainly see that part of the CFO’s remit. It sits within Digitise in our five-part model:
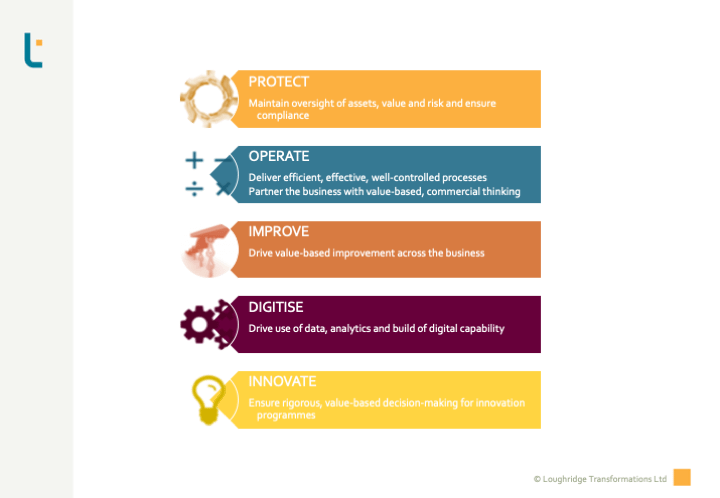
Data Science for Finance
But how do you go about doing that? And what do we mean by Data Science? Understanding the principles and applications of data science is crucial in leveraging its power in Finance.
What is Data Science?
Data Science uses statistical methods to understand the problems of Finance and other process areas. It combines the traditions of econometrics with the technological components of data science. Machine learning and predictive and prescriptive analytics can provide strong possibilities for better understanding financial data and solving related problems.
It includes techniques such as:
- Risk Analytics
- Quantitative Methods
- Hypothesis Testing
- Linear Regression
- Volatility Estimation
- Time-Series Analysis
- Simulation Methods
- Valuation
- Data wrangling
- Machine Learning Models
- Deep Learning Models
- Programming languages like SQL and Python.
The list can be even more extensive, depending on how complex the analysis is required to solve the problem.
Applications of Data Science in Finance
We can use Data Science within Finance in several ways.
Some real-life examples include fraud prevention, risk management, credit allocation, customer analytics, algorithmic trading, loan approval, and many more. When we apply data science to Finance, the unique combination helps build systems and processes and the required skills and behaviours that extract insights from financial data in various forms. It is a proven fact that Data Science has significantly improved risk analysis and anomaly detection, leading to notable improvements in detecting fraudulent transactions and money laundering activities.
Fraud Detection
A widely known application is Fraud Detection. Traditional fraud detection uses rule-based models to identify unusual transactions. Unfortunately, these models often flag legal transactions based on “broken rules” when millions of transactions co-occur. By contrast, machine learning creates algorithms that process large datasets with many variables to find hidden correlations between user behaviour and the likelihood of fraudulent actions.
Let me give a real-life example. A few years back, I had a Citi Bank Credit Card. One day, suddenly, I noticed two transactions of one dollar each in just one minute. I received the message that these transactions had been attempted, and the bank system blocked them automatically. Later, I called customer care and discovered that those transactions were fraud attempts from a remote location abroad.
The system identified my spending pattern. It perhaps knew my location, spending currency, and many more parameters, ultimately blocking the fraudulent transactions. This pattern identification is how a machine-learning model safeguards customers’ money. These days, banks are using data science for fraud detection and credit verification, expanding their customer base, credit augmentation, on-the-spot loan approval, and so on.
Customer Analytics
In customer service, forward-looking banks and FinTechs serve their customers better by analysing their transactional and behavioural data using various data science algorithms. Some of the world’s biggest banks are already using data science to gain insights into previous customer purchases, engagements, and accounts most relevant to them. For example, we all receive notices about investment products, insurance coverage, bank accounts, mortgages and other products that reflect our interests. Some people think it’s just a marketing gimmick. However, knowing our interests and targeting the specific customer segment helps those organisations increase profitability.
Deploying Data Science for Finance
Let’s now turn to how a Finance leader might implement some of the tools and techniques we have discussed. As a Finance leader, your role in implementing data science is crucial. You will be responsible for identifying the right tools and techniques, ensuring the necessary resources are in place, and guiding your team through the process.
At Loughridge Transformations, we use our Four Elements of Transformation model to diagnose and identify improvement opportunities and full-scale Finance Transformation.
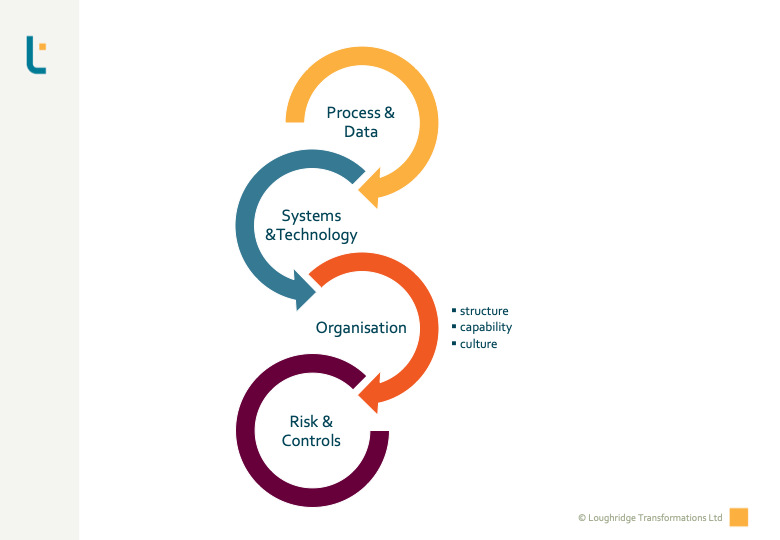
Our model shows the relationship between Process & Data, Technology, Organisation and Risk & Controls.
That relationship is equally valid when considering the application of Data Science. So, let’s explore some pre-requisites for creating value through Data Science.
Processes – what needs to be in place?
Let’s start by talking about Processes.
Imagine an organisation with very low process maturity. Process maturity refers to the formality and optimisation of processes within an organisation. Low process maturity means there is no actual process governance and limited attention to process performance and improvement, let alone an actively used process architecture. Unfortunately, many organisations still fall into that category today.
What can they do to leverage Data Science usefully? Or should that organisation first focus on implementing the basics?
First, it is crucial to create a long-term vision for analytics in the organisation and then back that vision with deep commitment and sponsorship. At the same time, it is critical to keep the evolving organisational environment and goals in mind. In addition, sustained investment in technology, resources, and training ensures enthusiasm and buy-in for analytics across the organisation.
If you have well-defined processes, they certainly complement the Data Analytics deployment. Conversely, the change management effort required is higher with lower process maturity. A lower level of process maturity usually means that the organisation is unfamiliar with process thinking, and at Loughridge Transformations, we believe that data analytics is part of process thinking.
However, that does not mean organisations will streamline their processes sequentially and then deploy Data Analytics. Most organisations still use analytics in a decentralised form, scattered across regions and organisational functions. As a result, Data Science might already be used ad hoc, in pockets, and without a proper strategy or roadmap.
Each organisation is unique, so it is vital to map out expectations from analytics at an organisation and project level. Let’s link this to Finance Transformation.
Suppose a Finance leader doesn’t have the relevant expertise or feel comfortable making these judgement calls. In that case, it can be a great idea to bring in external consultants like Loughridge Transformations, who have the expertise and experience to support the making of Return On Investment estimations and plan investments and deployments accordingly.
Pilot Projects for Data Science in Finance
So, let’s get very practical. It is critical to start small with a couple of pilot projects. After that, stay focused and then deliver quickly on these initiatives. It’s the same advice we give to all our clients who want to build a Data Analytics function or organisation.
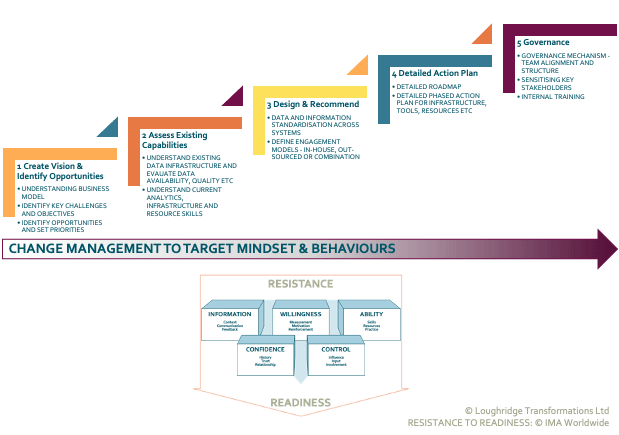
This approach helps provide visibility on analytics within the organisation and proves the potential value of deploying analytics. This visibility supports any proposals and increases the chances of stakeholder approval for more challenging or extensive use cases. It might not hurt to employ successful stories from analytics leaders by customising such solutions for one’s organisation and provisioning for differences in scale, organisation model, processes, and industry.
Now, how do we select the pilot projects? Project selection is a dilemma for most Finance leaders.
There could be many good candidates for finance processes, as we can apply data science to almost all finance processes. Still, it largely depends on the nature and scale of operations. For example, an FMCG organisation can deploy data science in its offer-to-cash vertical, which is a natural fit.
Record-to-Report could be another good candidate where we use Natural Language Programming and predictive analytics to drive future investment decisions or cost control measures.
Both Offer-to-Cash and Record-to-Report/Cost Management could be natural starting places, especially for larger organisations. However, there are also examples of creditworthiness that could be applied to customers, meaning organisations of any size can make more intelligent and agile decisions without increasing risk. Anywhere you want to identify root causes from trends in transactions that you cannot see with the naked eye, so to speak, or a bit of Excel magic. You can identify and fix value leakage, process inefficiency, control, or compliance issues from there.
Data – how about quality?
Many organisations struggle with data quality and even integrity. At Loughridge Transformations, we find that issue with almost any client with whom we have worked. So, dirty and/or inconsistent data quality could jeopardise any attempts to apply Data Science.
So, what should an organisation do if it has data quality concerns or has not yet implemented Data Quality Standards?
To apply data science, we need data for sure. And the logic is simple. Cleaner and more accurate data will produce better, more predictable results. But does it mean we can’t do anything if the data is scattered or the quality is patchy? No, that’s not the case. We can still start with something small. We can take smaller data sets, build focused models, solve minor problems, and eventually eat the big fish later. Of course, more significant leaps need better data quality; it is not a constraint to taking small baby steps.
Moreover, the Business Understanding stage is more crucial than whether the data are structured or unstructured. It is that stage that helps in defining the customer’s goal. In this stage, a data scientist has to ask the customer many questions about every aspect of the problem to clarify the organisational requirements. These requirements further define the data we need and how to collect it.
These days, text or numbers are not the only data. For example, even motion or a picture is data, and Data Science can also analyse such unstructured data.
Data quality, or lack thereof, is not a real showstopper. Instead, be tactical and work with what you have if you believe some value can be unlocked.
Organisation – how do you design roles and recruit candidates?
Let’s now move to Organisation Design. In addition to considering overall organisational structures, at Loughridge Transformations, we also consider the skills and behaviours required when working on a role and, ultimately, job design.
These days, we talk about human and machine-based roles – especially in some of Finance’s more heavily transactional processes.
Considering the human roles when it comes to data, we group the skills into three buckets:
- data intuition
- data transformation
- data communication or storytelling
Data Intuition
Here, we mean being able to interpret and use analytics to support decision-making. So, what skills should Finance leaders look for when hiring or developing staff?
Data Scientists are professionals who turn data into information, so statistical know-how is at the forefront of the toolkit. A data scientist’s core task is knowing the algorithms and how and when to apply them.
The second attribute of a Data Scientist is technical acumen. A natural interest in coding and understanding the logic of coding structure are essential.
And last but not least, it includes telling a story from the analysis. The data scientist must interpret and communicate the algorithmic output to decision-makers.
In short, a Data Scientist should be techno-functional, also called “Purple People”.
In a business context, “purple people” are those with the knowledge and insight to leverage new and emerging technologies and use data analytics to solve organisational problems.
However, many Finance Leaders who learned their trade in a time before Data Science may find it uncomfortable to recruit Data Scientists. Furthermore, it can be challenging to identify talent and expertise outside your core area.
The reality is that before bringing these skills in-house, a Finance Leader should work with a specialist at an earlier stage. They would have the knowledge to draw upon at opportunity identification and subsequent deployment.
Data Transformation
Data Transformation includes collecting and then using the data in the models (whatever mathematical basis) to prepare for the next step.
It is the foundation of Data Science in Finance and every domain. Unfortunately, we may not always get the desired format or structure of data needed for the correct analysis. But let’s understand what Data transformation is. It is all about changing the format of data, its type, structure, or values, cleaning the data, treating null values, looking out for duplicate entries at times, and even removing irrelevant columns or records.
The tools and techniques depend on the format, complexity, structure, and volume. During transformation, we do what we must do based on the organisational problem and the analysis we need to perform. For example, if we do aggregated analysis, we transform the raw data to show average, minimum, maximum, sum, and count statistics.
If we do attribute or feature construction, new attributes are constructed and added to the given set of attributes to help with data mining.
There are many other techniques for Data Transformation—the method is based on the analysis needed and the problem to be solved.
Let’s emphasise that Finance Leaders should not start with recruitment but first understand the problem and the analytical skillset needed. Recruitment can only come at a later stage in deployment. Organisations should experiment with proof of concept before making a heavy investment.
Data Communication
Lastly, we have data communication. At Loughridge Transformations, we say that data needs to become information to generate insights and finally make the intervention in the business. So whether we are talking about using statistics in Lean Six Sigma or any of the econometric or quantitative methods we mentioned above, storytelling is a fundamental component of being a high-performing Finance Business Partner. As Data Scientists, Lean Six Sigma Master BlackBelts or whatever the role is, we play with data and understand numbers. We use mathematical and statistical tools to arrive at some patterns or solve riddles where the answers are hidden in large and complex data volumes.
However, most of the time, Process Owners or senior stakeholders who ultimately decide for the organisation are not statistical or machine-learning model experts. They are just looking for solutions in simple language. As Data Scientists, we need to provide them with insights and recommendations in the storyline related to their organisational environment.
Many data scientists are great coders but lack communication skills or cannot articulate numbers in a story. That is often why great machine-learning models fail in deployment. Whether in Finance or any transformation journey, a data scientist must translate the numbers into a solution and communicate effectively with the decision-makers.
Risk & Controls – application of Data Science
The big thing in controls is moving from preventative and detective controls to predictive controls and risk analytics. This area lends itself to the use of Data Science, and as in some of the use cases we have cited, the Finance Services sector has been a relatively early adopter. However, the application is much broader than Financial and Non-Financial Controls in all industries. The starting point is a standard control framework with a good risk/control cycle, including proper procedures for testing design and operating effectiveness.
Indeed, we can hardly talk about Data Science applications in Finance without mentioning Risk Management. Data Science can mine past patterns and make specific predictions for the future. We can use these predictions, supported by evidence and deep analytical findings, to evaluate risks.
Some of the real-life used cases are in Identification of Exposure of Loss. Recording repeated risks can be of great significance to the organisation. With the help of this data, they can have a clear picture of the repetitiveness and severity of the risk involved. Data Science can help us better understand past occurrences of the incident. It can also clarify the consequences for the organisation and how other organisations have been affected.
The most significant aspects of Data Science in Risk Management are studying and preparing for future risks, considering past events, and understanding current scenarios. This approach results in more detailed and comprehensive decision-making for the organisation.
Need More Support on Data Science Deployment in Finance?
Has this whetted your appetite for leveraging Data Science in your organisation? If you need support, we can provide as little or as much as you need to get going or dig deeper, process your findings and pilot projects – including fit-for-purpose design, testing and implementation. So don’t hesitate to get in touch!
Alternatively, take a look at our most popular blog posts:
Or are you looking for something else? Here’s what we have been blogging about recently:
Agile Analytics Associates Automation Behaviours Building Trust Business-Partnering CFO Remit Change Management Coaching Collaboration Continuous Improvement Control Design Corporate Governance Data Deployment Design Principles Digital ERP ESG Finance Function Finance Transformation Implementation Migration Off-Shoring Organisation Organisation Design Process Process Design Process Governance Process Improvement Process Performance Productivity Project Management Readiness Risk & Controls Skills sponsorship Standard Organisational Model Strategy Systems Systems Design Technology Transformation Virtual Working
3 thoughts on “Unlock the Value of Data Science: Make or Break?”
Comments are closed.